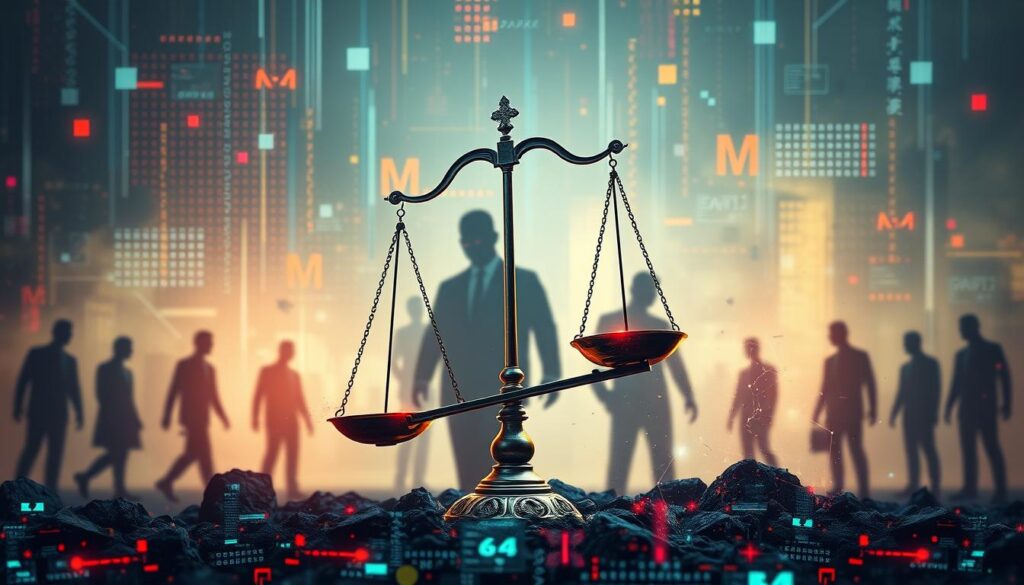
The digital world is changing fast, and generative AI is at the heart of this change. As we look into AI safety, a big question pops up: Are we ready to use this powerful tech right?
The risks of generative AI go way beyond just new tech. These systems, built from huge digital datasets, raise big questions about privacy, fairness, and how they affect society. Issues like bias in decisions and data privacy are huge concerns.
Getting to grips with these risks isn’t just about knowing tech—it’s about protecting our future. As AI touches every part of our lives, from jobs to creativity, we need a smart way to use AI ethically.
Table of Contents
Understanding the Fundamentals of Generative AI Ethics
Generative AI has changed technology fast, bringing new powers and big ethical questions. As you dive into artificial intelligence, knowing key ethical rules is key for making AI right.
The ethics of AI are complex and wide-ranging. Important things to think about include:
- Protecting individual privacy
- Ensuring algorithmic fairness
- Maintaining transparency in AI decision-making
- Establishing clear accountability mechanisms
Key Components of AI Ethics
Fixing AI biases needs a full plan. Companies must make strong rules to stop AI from being unfair. Ethical oversight is key to avoid bad outcomes.
“The true measure of AI’s success lies not in its technological prowess, but in its ability to serve humanity ethically and responsibly.” – AI Ethics Expert
The Evolution of Ethical Concerns
Generative AI has changed how we see ethics. Studies show 69% of AI experts say we need better ethics education and practices fast.
Current Challenges in AI Ethics Implementation
Even with more awareness, making AI right is hard. Big hurdles include:
- Lack of clear rules
- Fast tech changes
- Complex AI decisions
- Not knowing long-term effects
As AI grows, we must think ahead about ethics. This is key to building trust and making sure tech helps humanity.
Addressing Critical Ethical Risks of Generative AI
Generative AI is changing many industries, but it also raises big ethical questions. Your company must know the key risks these advanced systems pose.
Data privacy is a major worry. Studies show that 83% of companies see data security as a big challenge with generative AI. Sadly, 62% of users don’t know how their data is used by AI, leading to a big gap in transparency.
“Ethical AI is not just a technical challenge, but a fundamental human responsibility” – AI Ethics Expert
Some major ethical risks include:
- Potential data misuse and privacy violations
- Unintended bias in AI-generated content
- Lack of complete accountability measures
- Potential for harmful content generation
To tackle these risks, AI value alignment is key. Companies must take steps to ensure AI is used responsibly. This means setting clear rules, being open about how AI works, and always checking AI’s outputs.
The world of generative AI ethics is complex. With 67% of companies facing more AI-related cyber scams, your approach to ethical AI must be thorough and flexible.
It’s vital to understand and tackle these risks for responsible AI use. By focusing on ethics, you can use generative AI’s power while keeping individual rights and societal values safe.
Bias and Discrimination in AI Systems
Generative AI systems face big challenges in avoiding biases in many areas. The risk of discrimination is hidden in the algorithms that run today’s AI.
AI safety worries come from many sources of bias. These biases can greatly affect how AI makes decisions. It’s key to understand these biases for responsible AI use.
Types of AI Bias
- Data Bias: Happens when training data doesn’t show a wide range of people
- Algorithmic Bias: Comes from the design flaws in AI models
- Interaction Bias: Forms through how users interact and give feedback
Impact on Decision-Making Processes
Biased AI can pose big risks in important areas like:
- Hiring
- Financial lending
- Criminal justice
- Healthcare
About 70% of AI projects fail because of ethical worries about bias and discrimination.
Strategies for Bias Mitigation
Your company can take several steps to tackle AI biases:
- Do regular checks on AI algorithms
- Make training data more diverse
- Use machine learning that focuses on fairness
- Set up clear AI rules and oversight
By tackling AI safety issues head-on, you can make AI systems fairer and more reliable. This helps reduce unfair outcomes.
Privacy and Data Protection Concerns
Generative AI is a powerful tool with big privacy issues. As more companies use AI, keeping data safe is key. A recent survey found that 90% of companies focus on keeping data private when using AI.
AI privacy risks are more than just collecting data. There’s a big chance of misuse. Here are some main areas of concern:
- Identity theft through AI-generated deepfakes
- Unauthorized personal data exposure
- Sophisticated social engineering attacks
- Potential intellectual property breaches
“The sophistication of AI tools creates unprecedented challenges in maintaining individual privacy and data security.” – Digital Privacy Expert
AI security issues are a big worry. About 55% of people are concerned about how companies use their personal info. Insider threats cause 76% of data breaches, showing the need for strong protection.
To tackle these risks, companies should:
- Use strict data anonymization methods
- Set up clear consent rules
- Be open about how they handle data
- Invest in privacy tech
It’s not just a good idea to focus on AI privacy—it’s a must for keeping users’ trust and avoiding legal trouble.
Transparency and Accountability in AI Development
AI technologies are changing fast. It’s key to understand how they work. The complexity of AI models makes it hard to be transparent and accountable.
AI experts say we need clear systems. A survey found that 71% of AI professionals want better accountability. They want AI to be easier to understand.
Building Transparent AI Systems
To make AI clear, we need smart plans. Here are some ways:
- Use Explainable AI (XAI) techniques
- Make detailed documentation
- Set clear guidelines for model interpretation
- Build interfaces that explain AI easily
Establishing Accountability Frameworks
Being accountable in AI means having rules. Studies show that using XAI can make users 25% happier.
“Transparency is not just a technical challenge, but a fundamental ethical imperative in AI development.” – AI Ethics Research Group
Documentation and Reporting Requirements
Good AI governance needs thorough records. NIST suggests four main principles for AI explanation:
- Clear explanation capabilities
- Meaningful interpretations
- Explanation accuracy
- Understanding system knowledge limits
By focusing on transparency and accountability, we can make AI more reliable. This way, AI can help society and reduce risks.
Environmental Impact and Sustainability Considerations
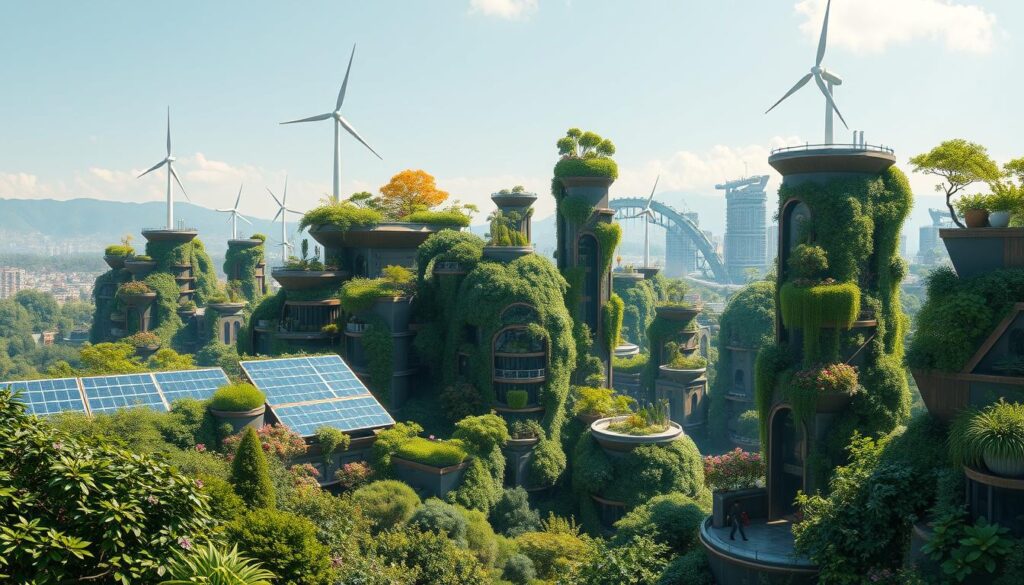
Generative AI’s risks go beyond just how well it works. We must think about its impact on the environment. These technologies use a lot of energy, which is bad for our planet.
The environmental impact of AI is huge. Here are some key points:
- Training an AI model can generate carbon emissions equivalent to approximately 300 round-trip flights between New York and San Francisco
- Data centers are projected to consume 8% of US power by 2030, a dramatic increase from 3% in 2022
- Global datacenter electricity consumption reached 460 TWh in 2022
“The environmental cost of AI innovation is not just a technological challenge, but an ecological imperative.” – Global Tech Sustainability Report
We need new ways to make AI less harmful to the environment. New ideas are coming up, like:
- Energy-efficient algorithms
- Green data center design
- Carbon-aware computing architectures
- Optimized machine learning models
Big tech companies are working hard to be more green. Microsoft is making AI models use less energy. Google is making chips that use less power but work better. This shows they’re serious about helping the planet.
Implementing Responsible AI Governance Frameworks
AI governance is complex and needs a strategic approach. It must balance innovation with ethics. As AI changes industries fast, companies must have strong AI governance to ensure responsible AI.
Only 10% of companies have a full policy for generative AI. This shows the urgent need for good governance strategies. Your company can be a leader by adopting effective AI governance practices.
Key Elements of AI Governance
Good AI governance frameworks should have:
- Clear ethical guidelines
- Risk assessment protocols
- Stakeholder engagement strategies
- Continuous monitoring mechanisms
Best Practices for Implementation
To ensure responsible AI, follow these key steps:
- Create cross-functional AI ethics committees
- Develop transparent decision-making processes
- Implement regular AI system audits
- Foster a culture of ethical AI awareness
85% of organizations believe an accountability framework could enhance stakeholder trust and reduce legal liabilities.
Monitoring and Evaluation Methods
Effective AI governance needs ongoing evaluation. Companies using the NIST AI Risk Management Framework see a 40% drop in compliance issues over three years. Your monitoring strategy should include:
- Periodic performance assessments
- Bias detection protocols
- Transparent reporting mechanisms
- Adaptive policy updates
By focusing on AI governance, you can make AI solutions that protect both your company and society.
Legal and Regulatory Compliance in AI Development
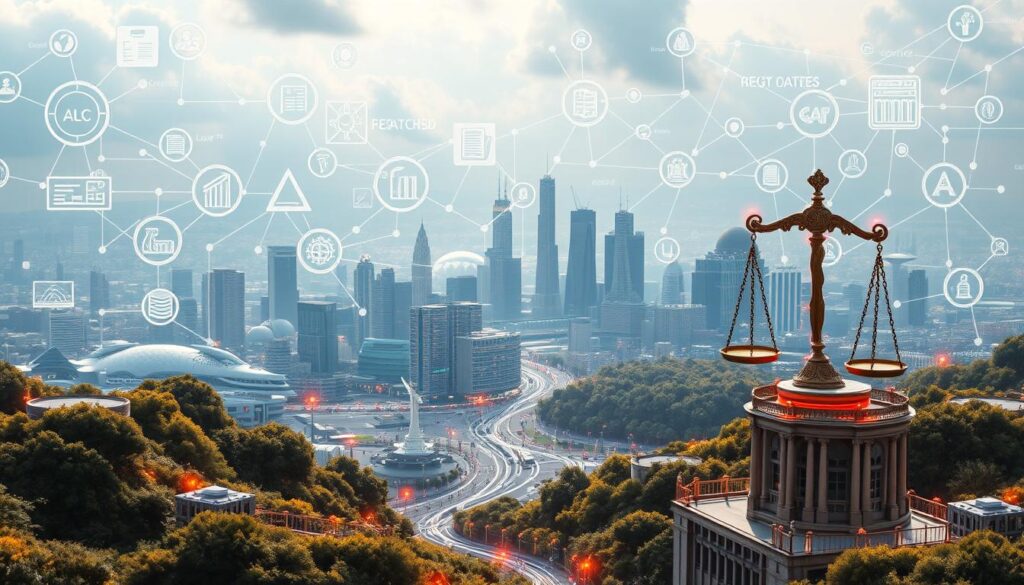
Understanding AI legal frameworks is complex. It involves tackling AI security and accountability. Generative AI’s fast growth poses big challenges for global regulators.
“Every two months, computers can do something we’ve never been able to do before.” – Kevin Weil, OpenAI
For AI developers and companies, knowing the legal landscape is key. Important regulations include:
- The European Union’s AI Act, proposed in 2021
- The Biden-Harris administration’s AI Bill of Rights
- NIST guidelines for AI risk management
Your company must focus on several key areas for compliance:
- Data Privacy Protection: Follow GDPR and CCPA rules
- Stop AI from being biased
- Make AI decisions clear
New laws require AI to meet standards by December 1, 2024. Only 43% of Americans trust AI. Strong legal frameworks are vital for trust and avoiding legal issues.
To stay compliant, conduct AI audits often. Use ethical data and clear AI tools. Responsible AI development helps avoid legal problems and encourages innovation.
Conclusion
Reflecting on generative AI, we see its ethical risks go beyond tech issues. To develop AI responsibly, we need a balanced approach. This means combining innovation with human values. With 63% of Americans worried about AI bias, it’s key for companies to align AI with values to gain trust.
Addressing ethical concerns is essential. Companies must create strong frameworks to tackle biases and protect privacy. They should also ensure transparency. This involves more than just tech fixes; it’s about integrating ethics into AI development.
Our commitment to ethical AI is a must, not just a tech rule. By focusing on education, setting high standards, and being open, we can create AI that respects humanity. The future of AI relies on our collective effort to navigate these complex issues wisely and with empathy.
As AI grows, so do our ethical duties. We must keep learning, adapting, and talking openly about these issues. By doing so, we can make sure AI benefits society, not harms it.
FAQ
What are the primary ethical risks associated with generative AI?
The main risks include bias and discrimination, privacy issues, and lack of transparency. There’s also the risk of AI misuse and ensuring AI aligns with human values. These issues affect many areas and can impact fairness and decision-making.
How can bias in AI systems be effectively mitigated?
To reduce AI bias, use diverse data and fairness techniques. Regular audits and inclusive teams are also key. Training and testing are essential to catch and fix biases before AI is used.
What are the key privacy concerns with generative AI?
Privacy worries include unauthorized data use and personal info exposure. Synthetic content could lead to identity theft. Keeping data safe is a big challenge with generative AI.
Why is transparency important in AI development?
Transparency builds trust and ensures accountability. It lets people see how AI works and spot biases. This is vital for ethical AI use.
What are the environmental impacts of generative AI?
Generative AI uses a lot of energy and causes carbon emissions. It also uses a lot of water. We need to make AI more sustainable.
How can organizations implement responsible AI governance?
Responsible AI governance means setting ethical guidelines and having oversight committees. It also involves risk assessment and continuous monitoring. Being adaptable to new AI and ethics is important.
What legal challenges exist in AI development?
Legal hurdles include navigating complex rules and liability issues. There’s also the need to keep up with new regulations like the EU AI Act. Rapid tech advancements make this hard.
How can AI systems be made more accountable?
To make AI accountable, use explainable AI and keep detailed records. Create audit trails and define responsibilities. Independent reviews help ensure AI systems are fair and transparent.
What role do stakeholders play in ethical AI development?
Stakeholders offer diverse views and conduct independent reviews. They provide guidelines and participate in governance. Their input ensures AI is developed with societal impacts in mind.
How can individuals protect themselves from AI-related risks?
Stay informed about AI and know your rights. Be careful with personal data and use privacy tools. Support ethical AI development and advocate for transparency.